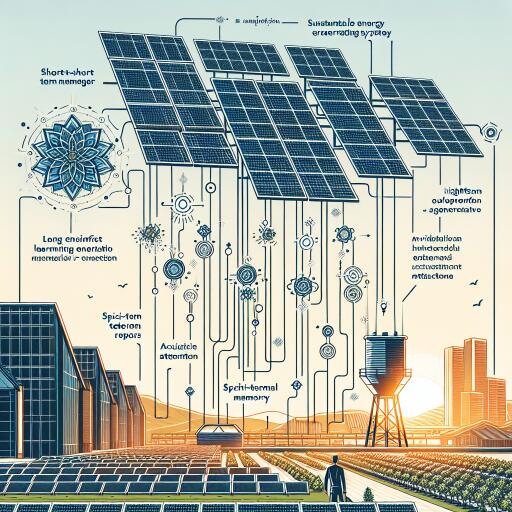
Revolutionizing Solar Powered Irrigation with Advanced LSTM Models
In the realm of renewable energy, the quest for efficient and cost-effective solutions to harness solar power for agricultural irrigation has taken a significant leap forward. Solar-powered irrigation systems, particularly in remote and underserved areas, stand as a testament to innovation in the face of necessity. These regions, often devoid of conventional electricity grid access, have found a promising solution in photovoltaic (PV) energy, capitalizing on the abundant solar radiation available in many parts of the world. The transformation of solar energy into electrical power through PV cells to run water pumps illustrates a sustainable approach to agriculture, a critical component for crops’ irrigation needs.
Nonetheless, the variable nature of solar energy generation presents a challenge, particularly when integrating into wider power systems aimed at consistent and reliable power supply. The development of precise forecasting models for PV energy output becomes critical. Such advancements not only enhance the efficiency of solar power utilization but also fortify the grid’s resilience, accommodating the unpredictable character of renewable energy sources.
Recent strides in technology have seen the solar power industry evolve, marked by a significant reduction in production costs. This evolution has positioned solar energy as a formidable competitor in the energy market, encouraging the proliferation of large-scale installations with capacities reaching hundreds of megawatts. Parallelly, the advent of smart metering in solar installations has enriched data collection, enabling the detailed analysis and prediction of solar energy generation through time-series data.
On the forecasting frontier, the reliability of predicting PV energy output hinges on integrating advanced modeling techniques. Among these, machine learning (ML) and deep learning (DL) models have shown exceptional promise. These models navigate through enormous datasets, identifying patterns and predicting outcomes with remarkable accuracy. In particular, the deployment of Long Short-Term Memory (LSTM) networks, a form of recurrent neural network, has provided a framework for capturing the temporal dynamics of solar energy production.
The integration of a spatio-temporal attention mechanism with LSTM presents an innovative approach to forecasting solar power. This model uniquely adjusts its focus on different time intervals, considering both past and future data to make predictions. The inclusion of spatial features — such as climate variables and power usage statistics from a connected irrigation system — enhances the model’s predictive accuracy. Through rigorous testing and analysis, the spatial-attention-based LSTM model has demonstrated its superiority, offering refined predictions that outmatch conventional ML and other DL models.
This attention mechanism offers a more nuanced interpretation of data, directing the model’s focus to specific time and spatial features that significantly impact energy generation. Its effects are profound, improving not only the accuracy of predictions but also the robustness of the forecasting model. Through comparative analysis, this innovative approach sets a new standard, markedly outperforming established prediction techniques across several key metrics.
The advancements in LSTM modeling for solar power forecasting present a promising avenue for enhancing the efficiency and reliability of solar-powered irrigation systems. By providing a more accurate prediction of energy generation, farms can optimize their water usage, improving crop yield while conserving precious resources. This leap in predictive capability represents a cornerstone in the quest for sustainable agriculture practices, marrying the need for environmental stewardship with the indispensable task of feeding the globe.
As this technology continues to evolve, its implementation could revolutionize not only agricultural irrigation but renewable energy’s role in creating a more sustainable, efficient world. The fusion of eco-friendly power with cutting-edge predictive models opens up new horizons for renewable energy applications, setting the stage for a future where clean energy and precision agriculture go hand in hand.
Leave a Reply