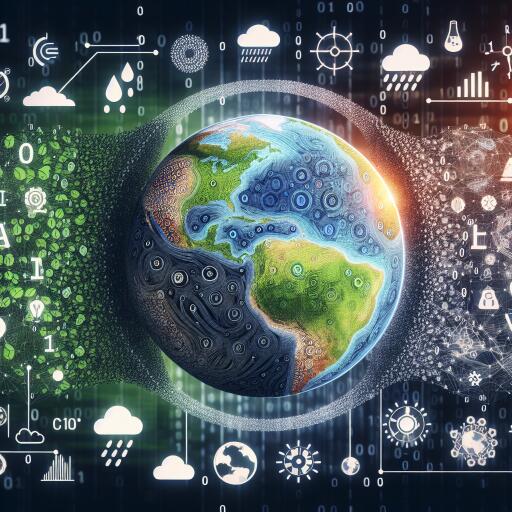
Machine Learning: A Game-Changer in Climate Prediction
The intricate challenge of precisely modeling clouds and determining their atmospheric heating and moistening contributions in climate prediction models has been a long-standing hurdle. This complexity contributes to the considerable variance present in current climate forecasts. Yet, making accurate predictions about how global temperatures will rise with increasing greenhouse gas levels is crucial for decision-makers worldwide.
Recent research unveils a promising advancement in addressing this issue through machine learning techniques. Scientists have demonstrated the potential of these techniques to enhance the representation of clouds in coarse-resolution climate models, approximately 100 km in resolution, thereby reducing the prediction range.
“We have large uncertainties in our prediction of the response of the Earth’s climate to rising greenhouse gas concentrations. The primary reason is the representation of clouds and how they respond to a change in those gases. Our study shows that machine-learning techniques could be revolutionary in enhancing cloud representations and thus improving forecasts of both global and regional climates.”
Employing an innovative approach, the researchers utilized an idealized model known as an aquaplanet—essentially a planet with no continents—to test their new method of convective parameterization using machine learning. They developed a deep neural network trained on simulations that explicitly model clouds, resulting in a machine-learning representation humorously dubbed the “Cloud Brain” (CBRAIN). This tool skillfully predicts a range of key climate simulation characteristics, including cloud-induced heating, moistening, and radiative features.
This methodological advancement hints at a promising future where the design of climate models can be data-driven and constructed from a “top-down” perspective. Such an approach involves discerning the significant features of complex processes and using them to inform model structures. Because the sensitivity of global temperature to carbon dioxide levels is closely linked to cloud representation, the integration of CBRAIN into climate models shows promise in improving temperature forecasts.
Furthermore, CBRAIN has been integrated and tested in fully coupled climate models, yielding highly encouraging outcomes. These models demonstrated improved capability in predicting responses to rising greenhouse gases. This innovation could indeed forecast climate dynamics with greater precision and reliability, offering valuable insights for policymakers and scientists alike.
The exploration of machine learning in weather and climate modeling represents a transformative movement in the scientific community. These new tools can perform on par with traditional methods while using substantially less computational power, a significant advantage as we push the boundaries of prediction accuracy. By merging artificial intelligence capabilities with traditional meteorological techniques, scientists aspire to revolutionize the realm of weather and climate forecasting.
The growing intersection between artificial intelligence and environmental science offers a beacon of hope for evolving how climate predictions are made. This technological evolution could radically transform our understanding and predictive abilities concerning one of the most pressing global issues—climate change. The implications are vast, opening doors to more precise long-term forecasts that could inform efforts to mitigate the impacts of our changing climate.
In summary, the integration of machine learning within the domain of climate science is charting a new course towards more accurate and reliable predictions. As these techniques continue to evolve, they hold the potential to reshape the landscape of climate modeling and forecasting, bringing invaluable insights to the forefront of environmental policy and decision-making.
Leave a Reply